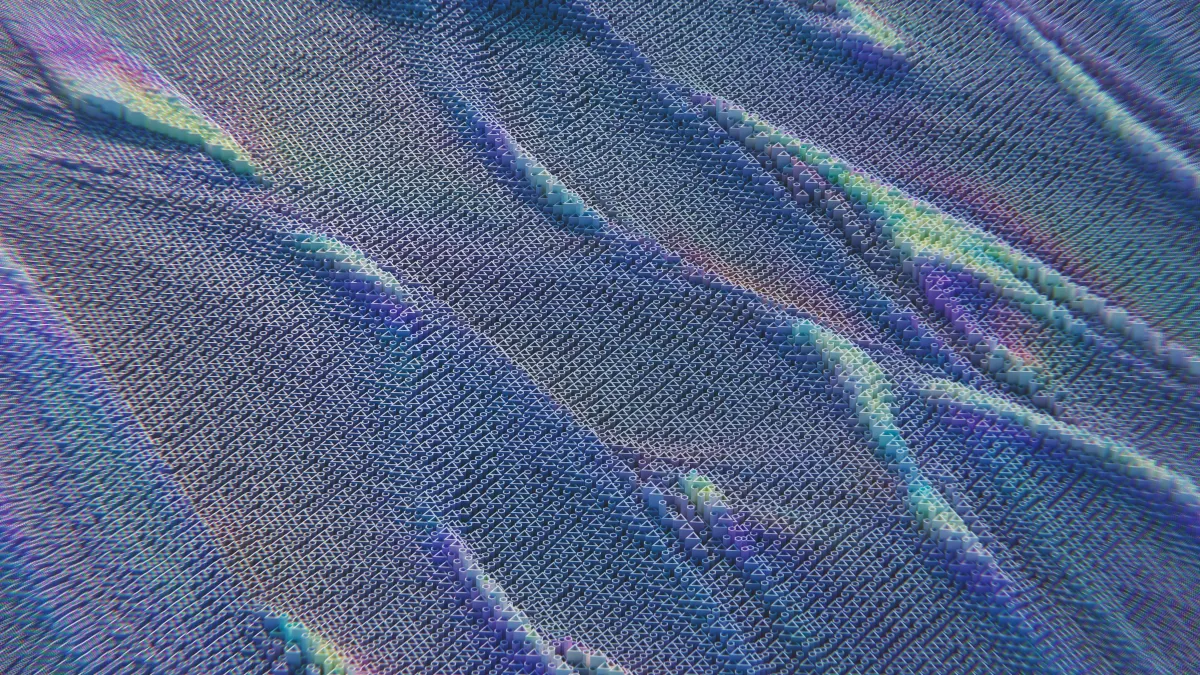
Barriers to AI Adoption Among Canadian SMBs
Barriers to AI Adoption Among Canadian SMBs and How to Overcome Them
This report was written with the help of AI
Artificial Intelligence (AI) promises to boost productivity and innovation, yet many of Canada’s small and medium-sized businesses (SMBs) have been slow to adopt AI solutions. This hesitancy is paradoxical given Canada’s strength in AI research and talent development – for example, Canadian institutions and companies are globally renowned in AI research – but much of the economic benefit has yet to be realized by SMEs on the ground. Over the past five years, Canadian businesses have consistently lagged in AI adoption compared to international peers. Studies indicate that only about 6% of small businesses and 8% of medium-sized businesses in Canada are using AI, versus 72% in the U.S. (with 35% of Canadian businesses overall using some form of AI). In fact, as recently as 2021, a Statistics Canada analysis found that only 3.7% of Canadian firms (with 5 or more employees) had deployed AI in any capacity – one of the lowest rates among advanced economies. This slow uptake spans across sectors from retail and services to manufacturing, even though AI applications (like chatbots in customer service, predictive maintenance in factories, or inventory optimization in retail) could greatly benefit these industries. Canadian SMEs’ cautious approach to new technology adoption – sometimes characterized as “risk-averse” or “fast-follower” behavior – has contributed to this gap.
Despite the lag, there are signs of change. The explosion of interest in generative AI tools in 2023–2024 (such as ChatGPT) has put AI on the radar of many businesses. Roughly one in seven Canadian businesses (14%) are now either using generative AI or plan to use it soon, with early adopters present in every industry. Larger firms and those in knowledge-intensive sectors have led the way – for instance, Ontario firms show the highest early adoption rates at 18%, and companies that export or have highly educated workforces are more likely to be experimenting with AI . Most small businesses, however, remain on the sidelines: nearly 73% of Canadian businesses have not even considered using generative AI yet as of late 2024. This report examines the primary factors preventing Canadian SMBs across all sectors from adopting AI solutions. It provides a brief historical context of the past five years and incorporates the latest insights (2024–2025) on key challenges – whether technological, financial, organizational, regulatory, or talent-related. It also draws on recent surveys and expert analysis to highlight these barriers with data, and proposes practical suggestions and policy-level solutions to help Canada’s SMEs catch up in leveraging AI for competitiveness and growth.
AI Adoption Trends (2019–2025): A Brief Overview
Over the last five years, Canada’s progress in AI adoption by businesses has been steady but modest, with SMBs significantly trailing larger enterprises. Early in this period, adoption rates were extremely low – Canada ranked only 20th out of 35 countries for business AI adoption in one 2021 benchmark. For example, in 2019–2020, surveys showed that over two-thirds of Canadian businesses had not identified any business need for AI at all, reflecting a widespread sense that AI was not immediately relevant to their operations. By the end of 2021, only a small single-digit percentage of Canadian firms had actually implemented an AI solution. This was despite increasing availability of AI tools and falling costs of computing – indicating that the barriers were more organizational and perceptual than purely technical at that time. Many companies were essentially in a wait-and-see mode, monitoring how AI played out elsewhere.
Entering 2022–2023, awareness of AI’s potential began to improve. The Canadian government and industry associations started new initiatives to encourage digital and AI adoption (for instance, the Canada Digital Adoption Program (CDAP) launched in 2022, and the expansion of the Pan-Canadian AI Strategy focused more on commercialization). By 2023, global surveys showed around 26% of Canadian organizations had at least one AI implementation, up from the prior years, but still below the global average (34%). The majority of Canadian firms were running only pilots or were in exploration phases. SMBs, in particular, remained far behind – one report noted just 6% of small businesses and 8% of medium businesses in Canada were using AI in 2023. In contrast, large Canadian enterprises (and U.S. companies of all sizes) were much more likely to have adopted AI, highlighting a growing digital divide. This “AI adoption gap” was deemed serious enough that in Budget 2024 the federal government announced a $2.4 billion package of AI-focused initiatives to boost adoption and commercialization. This included funding specifically to help SMEs adopt AI, recognizing that lagging adoption among smaller firms could hurt Canada’s overall economic competitiveness. By late 2024, two new federal programs were launched: the Regional Artificial Intelligence Initiative (RAII) – a $200 million fund to accelerate AI adoption by SMEs across sectors – and an AI Assist Program offering $100 million to support Canadian startups and SMEs integrating generative AI and deep learning into their products and services. These efforts underscore that the issue is not a lack of AI technology or talent in Canada, but rather translating those strengths into widespread use by businesses. In 2024, even with the buzz around AI, surveys found that only 32% of Canadian businesses expressed confidence in AI technologies, noticeably lower than the global average (39%).
In summary, while AI adoption among Canadian SMBs has inched upward in recent years, it remains in early stages. Most SMEs across retail, manufacturing, services, and other sectors are still not using AI in their day-to-day operations. The next sections explore why this is the case – the key barriers holding back AI uptake – followed by strategies to overcome these hurdles and ensure that businesses of all sizes and industries can participate in Canada’s AI revolution.
Key Barriers Preventing AI Adoption in Canadian SMBs
Adopting AI is not a simple plug-and-play upgrade for most small and medium businesses. It requires changes in technology, skills, budgets, and mindsets. Canadian SMEs face a combination of interrelated barriers that have slowed their uptake of AI solutions. Below, we outline the primary challenges – spanning technological, financial, organizational, regulatory, and talent domains – as identified by recent research and surveys. It is important to note that these barriers are common across sectors (whether it’s a retailer considering an AI-driven sales tool or a manufacturer exploring predictive analytics) though the severity of each barrier may differ by industry and firm size.
1. Lack of Awareness, Expertise, and Clear Use Cases
One of the most fundamental barriers is that many small business owners are unsure how AI applies to their business or whether it’s needed at all. In fact, a Statistics Canada survey found that over two-thirds (69%) of businesses that hadn’t adopted AI said it was because they had “no business need” for it. This points to a significant awareness gap – if decision-makers do not see a clear business case, they understandably will not pursue AI. A related finding is that 28% of non-adopters say they simply “don’t know what AI tools are available” or what AI could do for them. This unfamiliarity is especially acute for small firms that lack internal IT departments or R&D teams. Without knowledge of AI options, many SMEs cannot even begin to identify potential applications in their workflows. As the Business Development Bank of Canada (BDC) observed in a 2024 study, entrepreneurs are often “waiting for more concrete proof AI will help their business before investing”. In other words, until they see convincing evidence or examples of ROI, they hesitate to be first movers. This cautious attitude has been noted as a Canadian trait – experts cite a tendency toward risk aversion and a “fast follower” mentality, where companies prefer to see others go first.
The lack of AI literacy and expertise also means that many SMEs don’t know where to begin even if they are interested. Unlike large corporations that can analyze their operations for AI opportunities or hire consultants, small businesses often do not have a strategic roadmap for AI. As one Canadian report put it, “SMEs often lack the resources and expertise to implement AI solutions responsibly. Many lack the investments needed but even more simply do not know where to begin or have the talent needed to move forward.”. This leads to paralysis – without internal champions who understand technology or an outside push, AI projects never get off the ground. Notably, a recent Canadian Chamber of Commerce survey found that lack of internal knowledge (AI literacy) is a major hurdle, and businesses struggle to identify appropriate use cases for AI in their context. Even when AI tools are embedded in software they already use, some entrepreneurs don’t realize it – one BDC study revealed that 27% of Canadian small business owners were using AI-powered tools without realizing those tools involved AI. This unintentional use underscores the awareness issue: AI often remains an abstract concept rather than a concrete solution in the minds of many SMB owners. The outcome is that lack of awareness and unclear value proposition is the single largest factor behind low adoption – a barrier that must be addressed before others even come into play.
2. Financial Constraints and Uncertain ROI
Cost is a critical barrier for Canadian SMEs considering AI adoption. Unlike large firms, small businesses have limited budgets and must be very judicious with investments. Implementing AI – whether it’s purchasing software, procuring data, or hiring expertise – can be perceived as expensive. According to Deloitte’s analysis of Canada’s AI ecosystem, “financial costs and investments are the most common challenges to the growth ambitions of small and medium-sized [enterprises]”, even for those specifically interested in AI. In the context of ordinary SMEs (not just AI startups), this translates to difficulty affording the upfront costs of AI solutions or the infrastructure to support them. For example, acquiring an AI-driven analytics platform or customizing an AI application might require tens of thousands of dollars – a hefty sum for a small company if the returns are not guaranteed. It’s not just the software or tools – there can be hidden costs in data preparation, integration with existing systems, and training staff to use the new AI system. All these expenses make the business case harder to justify. It is therefore unsurprising that “implementation costs remain key issues” holding back entrepreneurs from investing in AI, as the BDC reported.
Another financial hurdle is the uncertainty of ROI (Return on Investment). Many SMEs worry that even if they invest in AI, the payoff is uncertain or long-term. Unlike buying a piece of equipment that clearly increases production capacity, the benefits of AI (e.g. improved decision-making, efficiency gains) can be harder to quantify upfront. Indeed, 69% of non-adopting firms said they haven’t identified a concrete business need or benefit (as noted earlier), which directly impacts their willingness to allocate budget. There may also be concern that AI projects could fail or that cheaper solutions might emerge later – essentially a fear of wasting money. Access to financing for technology projects is another aspect: smaller businesses might not easily obtain loans or investors for AI initiatives without a proven case. Approximately 30% of Canadian businesses surveyed cited access to financing as a top challenge in adopting new technologies (including AI). This indicates that even when an SME owner wants to adopt AI, they may struggle to secure the funds or credit to do so. In sectors like traditional manufacturing or retail with thin profit margins, there is often simply no slack in the budget for experimental projects.
Opportunity cost plays a role as well – money spent on AI might mean less money for other immediate needs (inventory, marketing, etc.). For many SMEs, maintaining day-to-day cash flow takes precedence over investing in unproven tech. Lastly, it’s worth noting that Canadian SMEs historically invest less in R&D and new technologies than large firms, contributing to a persistent productivity gap. This conservative spending culture is slowly changing, especially as success stories of AI-driven cost savings emerge. (For instance, entrepreneurs using AI report an average 27% reduction in operating costs, according to BDC’s findings.) However, until cost barriers are mitigated – through lower-price solutions or financial support – many SMBs will continue to shy away from AI purely due to budget constraints and ROI uncertainty.
3. Talent Shortages and Skills Gaps
Another major challenge for Canadian SMBs is the lack of skilled talent to implement and manage AI solutions. AI expertise is in high demand worldwide, and Canada’s tech talent often gravitates toward higher-paying opportunities in large tech companies or abroad, leaving a limited pool available for smaller businesses. A Canadian Chamber of Commerce report highlighted that roughly 3 in 10 businesses identified difficulty in hiring skilled employees as a top barrier to adopting new technologies like AI. Even if an SME is keen to use AI, they may simply not have anyone on staff who understands data science or machine learning. Hiring such specialists can be prohibitively expensive for a small firm; salaries for experienced AI professionals easily reach six figures. Furthermore, SMEs may not even know how to evaluate the skills of AI vendors or consultants, making it risky to outsource without internal knowledge. As a result, many SMEs face a capability gap – they lack both in-house expertise and the means to acquire it externally.
This skills gap extends beyond technical AI developers to the general workforce and management within SMEs. Effective AI adoption requires employees who can work with AI tools (e.g. interpret analytics, train an AI-driven system with proper inputs, etc.) and managers who can integrate insights from AI into decision-making. Currently, AI-specific training is rare in most small companies, and employees often learn on their own if at all. A 2023 survey of over 5,000 Canadian workers found that nearly 30% of employees report using AI tools at work, but 44% of these users have not received any formal training on AI. This implies that even when AI tools are present, many organizations (especially smaller ones) aren’t systematically developing AI skills among staff. The lack of training not only limits effective use but also raises risks (e.g. misuse of AI, security or privacy mistakes due to untrained users). It’s telling that only 21% of Canadian organizations feel confident in their ability to implement AI tools successfully – in other words, four out of five lack confidence, with small businesses being particularly unsure. This low confidence often stems from not having the right people or knowledge in place.
Moreover, Canada’s broad workforce has lower AI literacy compared to some peer countries. Global surveys reveal Canadians are generally less familiar with AI; many are “nervous” about it and rank low in self-assessed knowledge. If owners and employees don’t understand AI well, they are unlikely to champion its adoption. The competition for talent also means that an SME in, say, Atlantic Canada or the Prairies might find it nearly impossible to hire an AI specialist when big tech firms in Toronto, Montreal, or Silicon Valley are fishing from the same pond. This dynamic creates a talent bottleneck: even where there is budget and will to pursue AI, the human resources side lags. Until Canadian SMEs can access more talent – whether by training current workers or tapping into external expertise – their AI projects may stall. The talent gap, therefore, is a key barrier entwined with both the awareness issue and the execution of AI initiatives.
4. Organizational and Cultural Barriers
Beyond tangible resources, organizational culture and mindset within many Canadian SMEs pose a barrier to AI adoption. Small businesses are often lean operations focused on immediate needs; fostering an innovation culture can be challenging when time and resources are stretched. Many SMEs do not have a digital transformation strategy, and introducing AI might not be prioritized unless leadership explicitly drives it. In some cases, owners or managers may be skeptical of AI or uncomfortable with technologies they don’t fully understand. This cultural resistance can manifest as “let’s stick to what we know” attitudes. Indeed, Canadian business leaders have been described as cautious adopters – for example, only 32% of Canadian business respondents express confidence in AI technology, noticeably less than their American counterparts. Such lack of confidence often translates into organizational inertia; if management isn’t confident, projects won’t get approved.
Another cultural aspect is trust and ethical concern. AI systems, especially those that make autonomous decisions or recommendations, require a level of trust from the users. Both employees and customers might harbor concerns about AI – whether it’s fear of job displacement, biases in AI decisions, or data privacy. Canadians in general exhibit a wariness toward AI’s impacts. Surveys in 2023 ranked Canada 29th out of 31 countries in the share of citizens who believe products and services using AI have more benefits than drawbacks. In the workplace context, 70% of Canadian organizations voice concerns about the ethical implications of using AI and data tools. An SME’s team might resist AI adoption if they worry it could lead to layoffs or if they have moral reservations about, say, using AI on customer data. This means SME leaders need to manage change carefully – however, not all are equipped to do so, lacking change management experience. Without proactively addressing such concerns, companies may face internal pushback or lack of enthusiasm for new AI initiatives, undermining their success.
Additionally, implementing AI often requires cross-functional collaboration and new workflows, which can strain a small organization’s established routines. For example, a retailer adding an AI-driven inventory system needs its sales, purchasing, and IT (if any) to coordinate in new ways. These organizational adjustments can seem daunting. Employee acceptance is crucial; if staff don’t buy into the value of AI tools, those tools may go underutilized. Building that acceptance is part of organizational readiness. Unfortunately, many Canadian SMEs have not yet cultivated a pro-technology culture where experimentation is encouraged. They may also lack executive leadership with a vision around AI usage – something Deloitte identified as an important factor for an “AI-ready” culture. In summary, the soft issues of culture, change aversion, and trust form a barrier that is harder to quantify but very real. This barrier often interacts with others (for instance, a company with low awareness and skills will naturally be culturally hesitant). Overcoming it requires leadership, education, and often witnessing successful use of AI in a similar business to change minds.
5. Technological and Data Challenges
For SMEs that do attempt to explore AI, certain technological hurdles can impede progress. One issue is the challenge of finding or selecting the right AI tools and solutions. There is a rapidly expanding array of AI software, platforms, and vendors, which can overwhelm business owners who lack technical guidance. BDC noted that uncertainty about the “right tools” is a common barrier for entrepreneurs – they might be interested in using AI but unsure which specific technology fits their needs. This can lead to decision paralysis or wasted effort on trial-and-error with various tools. Moreover, some SMEs have legacy systems or poor-quality data that make AI implementation difficult. AI thrives on data; if a small business has not been digitally collecting data (e.g., still using paper records or very basic spreadsheets), there is little for an AI model to learn from. For instance, a small manufacturer might want an AI solution for predictive maintenance, but if their machines aren’t instrumented with sensors generating data, the project can’t even start. Incompatibility with existing systems is actually less frequently cited as an explicit barrier (only a small fraction of firms said technical incompatibility was the reason they haven’t adopted AI). However, technological readiness – having the IT infrastructure to support AI – is certainly a limiting factor. Many SMEs only began moving operations to the cloud or using advanced software in recent years, and AI adds another layer of complexity.
Another technical barrier is access to computing resources and data infrastructure. Training or running AI algorithms (especially advanced ones like deep learning) can require significant computing power, which small businesses don’t possess in-house. Cloud computing has made this more accessible, but there is still a knowledge gap in leveraging cloud AI services efficiently. Additionally, concerns around data privacy and security can make SMEs hesitant to fully utilize cloud-based AI (this ties into regulatory concerns addressed below). Data governance – managing and preparing data for AI – is a new competency that SMEs often lack. If a company’s data is siloed or unstructured, it can be a major project just to get it ready for an AI tool. This is why surveys find that the cost of necessary data preparation or new equipment registers as a barrier for some firms.
It’s also worth noting that some AI solutions on the market are tailored for enterprise-scale problems and may not fit the scale or simplicity needs of an SMB. A small retailer doesn’t need a complex AI supply chain optimization platform designed for a multinational – but without guidance, they might think AI is “too much” for them after encountering such products. Until recently, there were fewer AI products aimed squarely at small business use-cases, though this is improving now (with more user-friendly AI SaaS tools). Lastly, an often-overlooked challenge is that implementing AI is not a one-time event; it’s an iterative process. SMEs might try a pilot and get mediocre results (since AI models often need tuning or good data). Without in-house tech support, they may abandon the effort thinking “it didn’t work,” when in fact more iteration was needed. In summary, the technical complexity of AI – from selecting tools, readying data, to integrating into existing workflows – can be daunting for resource-constrained SMEs. This technical barrier intersects with the knowledge and talent gaps: a business with strong IT support can overcome it, but many Canadian SMBs are still developing that digital backbone.
6. Regulatory and Data Privacy Concerns
Though generally not the top barrier, regulatory and privacy concerns do play a role in AI adoption hesitancy among Canadian SMBs. Canada has stringent privacy laws (like PIPEDA) and sector-specific regulations (in healthcare, finance, etc.) that can make businesses cautious about how they use data and automated decision-making. Some small businesses worry that using AI might inadvertently violate privacy rules – for example, using customer data to train an AI system could raise compliance questions. In the BDC’s survey of entrepreneurs, “data privacy concerns” were noted as one of the barriers to AI adoption for SMEs. Particularly in sectors dealing with sensitive information (medical clinics, legal services, etc.), owners may shy away from AI that touches personal data due to fear of breaches or regulatory penalties. Even outside of privacy, there is a broader uncertainty about how AI might be regulated in the near future. The Canadian government is in the process of formulating the Artificial Intelligence and Data Act (AIDA), which will establish rules for AI systems (especially high-impact ones). While this law is still being refined, the prospect of new regulations creates a “wait and see” approach for some firms – they prefer to see the final rules before investing heavily, to ensure compliance requirements are clear.
That said, current research suggests that legal and regulatory issues are not the leading reasons for non-adoption at present. The Toronto Metropolitan University’s Dais research institute found that only 2% of non-adopting firms cited legal concerns as a reason for not adopting AI, and only 5% cited privacy or security concerns. These percentages are relatively low, indicating that while regulations and privacy are on the radar, they are typically secondary worries. (It appears that if a firm sees no business value or can’t afford AI, questions of regulation don’t even get that far in the decision process.) Nevertheless, for those SMEs that are more advanced in considering AI, issues like ethical use, bias, and liability do start to matter. For example, an HR firm might hesitate to use an AI resume screening tool out of concern that it could unintentionally discriminate and land them in legal trouble. Or a company might be unclear about intellectual property ownership of AI-generated content. Canadian businesses also pay attention to global standards – for instance, the EU’s AI regulations – and wonder if similar strict rules will come to Canada, which could constrain certain AI applications.
In addition, public trust and consumer expectations influence SMEs. If customers are uncomfortable with AI (say, an AI chatbot for customer service), a small business might hold off implementing it to avoid alienating their clientele. As mentioned earlier, Canadians are relatively cautious about AI, so a business might actually use the lack of customer demand or potential customer skepticism as a reason to delay AI adoption. In heavily regulated industries (like financial services, healthcare, transportation), even small firms must navigate complex compliance landscapes, which can slow down experimentation with new AI tools until those tools are vetted. In summary, while regulatory compliance and privacy are not the primary barriers for most SMEs, they form an important backdrop that can exacerbate other issues. Companies want assurance that they can adopt AI responsibly and legally. The call for “proportionate and risk-based” regulation – as recommended by the Canadian Chamber of Commerce – highlights the need to strike a balance so that necessary rules do not unintentionally stifle innovation among smaller players.
These barriers often compound one another. For instance, a lack of internal expertise (talent) makes it harder to assess which AI investments are worth the cost, or to navigate regulatory nuances, thereby amplifying financial and compliance concerns. Similarly, an unclear business case (awareness issue) makes a firm less likely to allocate budget (financial issue) or hire talent. The net effect has been a cautious stance among Canadian SMBs toward AI. However, recognizing these pain points is the first step toward addressing them. There is growing consensus among policymakers and industry leaders that these barriers can and must be overcome for Canada’s economy to fully benefit from AI. The next section discusses strategies and solutions – from both the policy level and practical business level – to help Canadian SMEs across all sectors adopt AI in a responsible and effective manner.
Strategies and Solutions for Overcoming AI Adoption Barriers
Accelerating AI adoption among Canadian SMBs will require concerted efforts on multiple fronts. There is no single silver bullet; instead, a mix of awareness initiatives, skill development, financial incentives, and supportive policies is needed to address the diverse barriers outlined above. Below, we present a comprehensive set of suggestions and solutions tailored to the Canadian context. These recommendations draw on expert commentary, successful case studies, and policy proposals, aiming to help SMEs in all sectors begin or expand their AI journey. For clarity, we organize the solutions into several categories: building awareness and know-how, easing financial hurdles, developing talent, fostering a supportive culture, and enabling policy measures. Many of these actions reinforce each other – for example, training programs improve awareness, and funding pilots helps prove ROI, which in turn builds awareness through success stories. The overarching goal is to create an environment where even a small retail shop in Toronto or a mid-sized manufacturer in Manitoba can confidently implement an AI solution that adds value to their business.
1. Building Awareness and Demonstrating Value
Education and outreach are paramount. Since a core issue is that many SMEs don’t see how AI can help them, a key solution is to show them. Industry associations, government agencies, and tech organizations should ramp up efforts to demonstrate practical AI use cases for SMEs. This can be done through workshops, webinars, or roadshows specifically targeting non-tech companies. For example, chambers of commerce and boards of trade across Canada could host events where local businesses that successfully adopted AI share their experiences and results. Seeing peers (say, a fellow mid-size manufacturer or a fellow retail chain) achieve cost savings or sales growth with AI can greatly reduce skepticism. The Canadian Business Data Lab and other research bodies are already providing valuable data; translating these into simple narratives (like “AI helped a small wholesaler cut inventory costs by 20%”) will help SMEs identify a business need for AI. The federal and provincial governments can collaborate on an AI awareness campaign for SMEs – similar to past initiatives in promoting e-commerce – highlighting how AI is not just for big tech, but for “Main Street” businesses too. Such outreach should also clarify what AI is, addressing misconceptions and hype, so that business owners have realistic expectations.
Additionally, promoting AI literacy is critical. Basic educational programs (online courses, short certifications) in AI for business should be made widely accessible and affordable. This could involve partnerships with community colleges or organizations like the Vector Institute or Mila (Canada’s AI institutes) to deliver AI literacy workshops for SME owners and managers. Improving general understanding will help leaders feel more confident about exploring AI. In fact, AI literacy for all Canadians has been identified as a foundation for adoption – a competency framework developed by researchers emphasizes the need for broad “AI innovation skills” and literacy, not just deep technical skills. Governments and industry groups should subsidize or sponsor such training, recognizing it as an investment in the country’s digital capacity.
Crucially, showcasing ROI and providing evidence of benefits can turn the abstract into concrete. Pilot projects and case studies play a big role here. One approach is for public programs or larger enterprises to sponsor pilot AI projects with willing SMEs. By lowering the risk and cost of initial trials (through grants or expert support), SMEs can experiment with AI on a small scale. If the pilot yields measurable improvements (e.g. an AI scheduling tool saves 10 hours a week in admin time), those results can justify further investment. Indeed, experts recommend a “start small” strategy: “begin with small-scale pilot projects to validate the feasibility and impact of AI before gradually expanding”. This agile approach lets businesses learn and build confidence. Organizations like BDC have begun to offer advisory services that help identify such pilot opportunities and guide implementation. The Business Development Bank’s “AI Imperative” report provides practical advice and even examples of common AI applications that SMEs can try in areas like cost control, sales growth, and labour savings.
Furthermore, creating communities of practice or knowledge-sharing networks can boost awareness. SMEs trust recommendations from peers and experts they know. Setting up an SME AI adoption mentorship program – where businesses that have implemented AI mentor those that are just starting – could be very effective. This can be facilitated by industry associations or regional economic development agencies. The goal is to ensure no small business feels it is charting unknown waters alone; instead, they have templates and success stories to follow. As more companies see real examples of AI driving value (whether it’s a service firm using AI to improve customer retention, or an agribusiness using AI for crop monitoring), the perceived lack of business need will diminish. Over time, the narrative should shift from “Why adopt AI?” to “How can we afford not to, when competitors are benefiting?”. In summary, robust efforts to educate, inform, and illustrate the value of AI will chip away at the awareness barrier that is currently so prevalent among Canadian SMEs.
2. Financial Incentives and Support Programs
Given that cost and risk are major concerns, another set of solutions revolves around financial support and incentives to make AI adoption more affordable for SMBs. The Canadian government has already recognized this need: as noted, Budget 2024 earmarked substantial funding to catalyze AI uptake among businesses. Building on this, there are several approaches to alleviate financial barriers:
Direct funding and grants: Expand and promote programs that offer grants or non-repayable contributions for SMEs implementing approved AI projects. For example, the new Regional Artificial Intelligence Initiative (RAII) provides $200 million to help bring AI to market and into SMEs. Ensuring this program is accessible to ordinary small businesses (not just tech startups) will be important. Similarly, the AI Assist Program ($100 million) supports SMEs integrating generative AI – this should be communicated widely so businesses know such help exists. At a smaller scale, reintroducing or augmenting programs like CDAP (Canada Digital Adoption Program) with a specific AI focus could help with micro-grants. CDAP’s “Boost Your Business Technology” stream, for instance, offered grants up to $15,000 for creating digital adoption plans – something like this could be tailored to AI strategy development for an SME. Some provinces may also consider matching grants or innovation vouchers that SMEs can use to offset the cost of AI software or consulting.
Loans and tax incentives: Low-interest loans or loan guarantees earmarked for technology adoption can encourage investment by reducing the financial risk. BDC and other financial institutions could create specialized loan products for AI and automation investments, complementing their existing tech loans. On the tax side, the government could provide accelerated depreciation or tax credits for AI-related expenditures (similar to how investments in manufacturing equipment often get favorable tax treatment). If an SME knows that, say, 40% of their AI project cost can be claimed as a tax credit, it might tip the scales toward a go decision. Canada already has the SR&ED (Scientific Research & Experimental Development) tax incentive program, which some AI development qualifies for; however, many small firms find SR&ED complex. Streamlining claims for AI adoption (not just R&D) or introducing a simpler “digital adoption tax credit” could be beneficial.
Shared resources and group buys: Another way to lower costs is by encouraging shared services models. For instance, a consortium of small businesses in the same region or sector could share the expense of an AI expert or a cloud computing resource. Government or industry associations could facilitate group licensing deals for popular AI tools, yielding volume discounts that individual SMEs couldn’t get on their own. There are precedents in other domains (e.g., small retailers banding together for group health insurance plans); similarly, groups of SMEs might jointly hire an AI implementation team that rotates among them. Public sector involvement can kick-start such collaborations by providing initial funding or coordination.
Proving ROI through funded pilots: As mentioned earlier, funding pilot projects is an investment that can pay off by demonstrating ROI, which then unlocks private spending. Programs could be structured to require a small cost-share from the business (to ensure buy-in) and cover the rest from public funds or corporate partners. If the pilot succeeds, the SME can then confidently invest their own money to scale it up. If it fails, the financial hit is cushioned. Over time, a repository of these pilot results (anonymized if needed) could be built to provide benchmark data on ROI for different types of AI use cases, further reducing uncertainty for others.
Importantly, awareness of funding is as crucial as the funding itself. Governments and banks need to proactively reach out to SMEs (not assume they will navigate bureaucratic websites on their own). Simplifying the application processes and providing advisory support to apply (perhaps via regional innovation centers or the Canada Business Network) will improve uptake of these financial supports.
Finally, private sector players have a role: large tech companies like Microsoft, Google, or Shopify that provide AI solutions can consider special pricing or mentorship for SMEs (some already do via their enterprise development programs). Public-private partnerships might extend such initiatives. The bottom line is that reducing the financial risk through external support can encourage more SMEs to take the leap. Canada’s approach of investing in SME adoption (the $2.4B package) is a strong start, and continuing to refine these incentives based on feedback will be key. If cost is neutralized as an obstacle – or at least significantly mitigated – many businesses will be far more willing to try AI, especially once they have the knowledge of what to try.
3. Developing Skills and Access to Talent
To address the talent and skills gap, a multi-tiered strategy is necessary – one that builds AI capacity within the existing workforce and also makes expert help more accessible to SMEs. Here are some key solutions on this front:
Workforce Upskilling and Training: Canada can ramp up initiatives to train existing workers and managers in AI-related skills. The Future Skills Centre, for example, has been supporting projects in this area. One noteworthy initiative is the Skills Bridge program developed by the Ontario Chamber of Commerce, Magnet, and TMU’s Diversity Institute. This program creates a shared platform with curated training and use cases specifically for SMEs, and connects them with student talent. Over 500 companies have participated, gaining access to AI and digital skills training they otherwise might not afford or even know where to find. Scaling up such programs across provinces (and continuing to fund them) will help thousands of SMEs build in-house capabilities. Online training modules, offered through partnerships with platforms like Coursera or LinkedIn Learning but subsidized for Canadian SMEs, could quickly raise the baseline of AI literacy for employees. Crucially, training should not only target technical staff, but also non-technical roles – salespeople, analysts, operations managers – so they can understand and leverage AI tools in their domain. Government grants or tax deductions for companies that invest in employee training in digital skills could incentivize more participation.
Talent Placement and Advisory Services: Bridging the talent gap can also be done by connecting SMEs with external experts in a manageable way. For instance, programs that place AI interns or fellows into small businesses for a few months can be highly beneficial. Organizations like Mitacs (known for linking academia and industry through internships) could have a stream focused on placing AI/data science students or recent graduates into SME projects, with the cost partially covered by the program. This gives the SME hands-on help while giving the student real-world experience – a win-win. Similarly, the federal government could sponsor a “Digital Adoption Advisors” program (much like CDAP did) where trained advisors visit SMEs to help them formulate an AI adoption plan or do initial setup. BDC’s advisory services and other consulting firms are available, but cost is often a barrier – subsidizing advisory for AI (even a few days of an expert’s time) could make a difference in kick-starting projects.
Leveraging AI Institutes and Innovation Hubs: Canada’s AI research institutes (Vector Institute in Ontario, Mila in Quebec, and AMII in Alberta) and the Scale AI supercluster are treasure troves of expertise. They are increasingly branching into industry adoption. More programs like AMII’s “AI Pathfinding” for businesses or Vector’s SME-focused initiatives can be encouraged. The idea is to open up these centers of excellence to mentor and guide SMEs. For example, an SME could apply for a mentorship where an AI researcher or engineer from one of these institutes helps the company identify a use case and prototype a solution over a few weeks. Scale AI already co-funds collaborative projects (particularly in supply chain AI) that often include SMEs; continuing and expanding such co-investment models will both reduce cost and inject expert knowledge into the SME. Additionally, universities and colleges could offer AI coop programs where students spend a term solving a data problem for an SME – essentially a structured way of transferring knowledge from academia to small business.
Immigration and Talent Attraction: On a broader policy level, ensuring Canada continues to attract global AI talent will indirectly help SMEs. If more AI professionals settle in Canada (through immigration programs or retention of Canadian graduates), some will eventually engage with or found SMEs. While a small company might not directly hire a senior AI scientist emigrating from abroad, a vibrant talent ecosystem creates more opportunities for knowledge spillover, contracting, or startup formation that benefits the SME landscape. Programs like the Global Talent Stream (which fast-tracks work permits for tech talent) should remain robust and perhaps emphasize roles in AI.
AI Tool Simplification (AutoML and No-Code): Another aspect of bridging the talent gap is promoting tools that lower the technical skill required to use AI. Many vendors now offer AutoML (automated machine learning) or no-code AI platforms. SMEs should be made aware of these, as they allow non-experts to implement certain AI solutions with a friendly interface. Encouraging SMEs to start with such tools (maybe through guided pilots) can bypass the need to hire a PhD in data science for simpler tasks. Over time, success with these tools can justify bringing in more advanced talent for more complex projects.
In essence, the solution to the talent barrier is to “bring the mountain to Mohammed” – i.e., bring AI expertise to SMEs through training or outreach, rather than expecting SMEs to somehow conjure experts immediately. By upskilling current employees and creating avenues for external expertise to plug into SMEs (temporarily or permanently), Canada can significantly boost the capacity of small businesses to adopt and sustain AI. Many of these efforts are already in motion via Future Skills Centre projects, industry training programs, and government pilot programs; the priority is to amplify and expand them so that they reach a critical mass of SMEs nationwide, in urban and rural areas alike. As more workers gain AI skills and more SMEs complete successful projects, a virtuous cycle can emerge where talent begets adoption, which in turn creates demand for more talent.
4. Fostering a Pro-AI Culture and Organizational Readiness
Overcoming cultural and organizational barriers requires leadership and change management initiatives that help SMEs build a more innovation-friendly environment. Here are some ways to do that:
Leadership Engagement and Vision: It often starts at the top. Educating SME leaders (owners, CEOs) on the strategic importance of AI is crucial so they can champion it internally. Short executive courses or bootcamps on “AI for Business Leaders” can be offered through business associations or local colleges. These would focus not on coding, but on how to integrate AI into business strategy and how to lead an AI project. If leaders develop a vision for how AI could improve their company (even in small ways), they are more likely to allocate resources and inspire their team to get onboard. We need more SME leaders to view AI not as a buzzword but as a practical tool – for example, to see it as “the next step in business process improvement” akin to past shifts like adopting the internet or data analytics. When top management clearly supports an AI initiative and communicates its importance, employees will be more receptive, and the organizational will is stronger.
Change Management and Employee Involvement: Implementing AI should be approached as a change management exercise, not just an IT project. This means involving employees early, addressing their concerns, and clarifying how their roles will interface with the new AI tools. Transparency and communication are key to building trust. If workers fear that an AI system will automate their job away, management should honestly address this – perhaps the AI is actually meant to remove tedious tasks so employees can focus on higher-value work. Offering reassurances (and backing them up with actions, like retraining for higher-skilled roles) will reduce resistance. In many cases, AI adoption in SMEs will augment staff rather than replace them, and framing it that way helps gain acceptance. As one Deloitte survey indicated, having “employee acceptance of AI’s importance” and confidence that AI won’t put jobs at risk are important elements of an AI-ready culture. Companies can foster this by celebrating examples where AI made someone’s job easier or enabled better outcomes, thereby positioning AI as a helper to staff, not a threat.
Ethical AI and Governance Frameworks: SMEs can borrow a page from larger organizations by establishing simple guidelines for AI use and data ethics. This might sound advanced for a small business, but even a one-page policy that says, for example, “we will ensure AI decisions are reviewed by humans when critical” or “we will protect customer privacy and be transparent if AI is used in communications” can build trust among employees and customers. Knowing that there is an ethical framework can make staff more comfortable engaging with AI systems. Canada recently launched the Canadian Artificial Intelligence Safety Institute (in 2023) aimed at ensuring ethical AI development. While its work will likely focus on broader policy, the awareness it creates can trickle down. SMEs can voluntarily follow emerging best practices for responsible AI. For instance, if an SME uses AI to screen resumes, they might periodically audit the outcomes for bias. These actions not only prevent negative outcomes but also improve confidence among stakeholders that AI is being used responsibly. It addresses the 70% of organizations concerned about ethical implications by proactively managing those implications.
Peer Networks and Culture Change: Sometimes cultural change is motivated by peer pressure or competition. If a few businesses in a community successfully adopt AI and gain an edge, others may feel encouraged to try (so as not to fall behind). Thus, creating local or sector-based peer networks for digital transformation can gradually shift the norm. When AI adoption becomes a more common topic of discussion among SME owners (e.g., at industry meetups or in trade magazines), it becomes normalized and even expected. This can erode the complacency that comes from thinking “none of my peers are doing this.” Government and industry bodies can facilitate such networks by funding meetups, case study publications, or innovation awards that recognize SME achievements in AI.
Time and Space for Innovation: Culturally, SMEs often feel they are too busy to experiment. One practical idea is encouraging businesses to allocate a small percentage of time or budget to innovation activities – often called “20% time” or an innovation budget. While it’s hard for a resource-strapped company, even dedicating a few hours a week for a staff member to explore AI tools, or setting aside a modest budget for trying a new software, can plant seeds. If needed, external programs could subsidize a temporary staff augmentation so the company can free up an internal person to focus on an AI pilot.
In summary, shaping a pro-AI culture in SMEs involves leadership buy-in, trust-building, employee empowerment, and embedding AI into the company’s values and practices in a positive way. It transforms AI from something foreign into a natural extension of the business. Overcoming fear and resistance requires empathy and education – showing employees that AI can make their work more interesting or effective, and showing owners that their business can remain competitive and even create better jobs by adopting technology. As more SMEs experience small “wins” with AI, confidence will build, and culturally the organization becomes readier to pursue further innovations.
5. Policy and Ecosystem Initiatives
At the policy level, Canadian governments (federal, provincial, and local) as well as the broader innovation ecosystem have levers to pull that can significantly accelerate AI adoption among SMEs. Many of these align with or complement the strategies already discussed:
Strategic National Targets and Coordination: The Canadian Chamber of Commerce’s Business Data Lab has recommended that Canada set ambitious AI adoption targets, such as aiming for 50% of businesses to adopt AI by 2030. Establishing such targets in official strategy (for example, including them in the federal Innovation Minister’s mandate letter) can galvanize action and resources. It signals long-term commitment and allows tracking progress year by year. A coordinated national AI adoption strategy would involve multiple ministries – not just innovation, but also those handling small business, finance (for incentives), education (for skills), etc., ensuring efforts aren’t siloed. This could mirror how Canada has strategies for broadband internet rollout or export growth, but focused on technological adoption.
Ensuring Proportionate Regulation: As new AI-related regulations are developed (like AIDA), policymakers should continue to consult with businesses and experts to ensure rules are clear and proportionate to risk. SMEs generally lack large compliance departments, so overly complex or burdensome requirements could inadvertently discourage them from using AI. A solution is to provide template compliance guides or even simple tools to help SMEs ensure their AI use is within legal bounds. For example, if algorithmic transparency is required in the future, a government website could offer a basic checklist or generator for an AI use transparency report. Also, regulators might consider safe harbor provisions for SMEs or phased implementation giving smaller firms more time to adapt. By making regulation SME-friendly, we can address legitimate ethical and safety concerns without creating an adoption chill.
Public Sector as a Lead User: Government itself can help SMEs by being an early adopter/customer of AI solutions from SMEs. Public sector procurement can include pilots where small Canadian AI firms or consortia are hired to implement solutions for government operations (with appropriate support). This not only gives those SMEs business, but it creates reference cases that can inspire others. A small company that sees a peer succeed in delivering an AI project for, say, a municipal government, might be more inclined to trust that similar solutions could work for them. Additionally, governments can open up more open data for SMEs to use in building AI solutions – for example, making datasets on economic trends, traffic, or health (with privacy safeguards) available can spur SME innovation in AI applications relevant to those fields.
Infrastructure and Platforms: The government’s planned “AI Compute Access” program and AI Sovereign Cloud/Compute Strategy is a forward-looking step. Ensuring that SMEs benefit from this – e.g., through cloud credits or shared access to high-performance computing for training AI models – will tackle the infrastructure barrier. The idea is to create national or regional platforms that SMEs can plug into, rather than each SME having to set up their own from scratch. Likewise, supporting the development of Canadian AI platforms or marketplaces where SMEs can easily find pre-trained models or AI services relevant to them (perhaps certified for quality or compliance) would reduce friction.
Industry Sector Initiatives: Different sectors have specific needs, so targeted programs can help. For instance, in manufacturing, the federal/provincial governments could expand programs like the Accelerated Supercluster Initiative (Scale AI is one such supercluster) which co-funds AI projects in supply chains and manufacturing. For agriculture, programs via Agri-Food Canada might promote AI in precision farming for small agri-businesses. In healthcare, grants to encourage small clinics or health startups to use AI diagnostics could be introduced, while ensuring privacy is maintained. Sector-focused innovation hubs (like Canada’s Digital Technology Supercluster in BC, etc.) often include SMEs; continuing to fund and include SMEs in their projects means those sectors’ smaller players aren’t left out.
Collaboration and Knowledge Hubs: Establish digital innovation hubs or AI labs in every region where SMEs can come for advice, training, and even try out AI technologies. Europe, for example, has a network of Digital Innovation Hubs that help SMEs with Industry 4.0 and AI adoption through “test before invest” facilities. Canada could do similarly via colleges or tech parks – a place with some data scientists and equipment where a business can experiment at low cost. This reduces the barrier of not knowing where to start or not having the environment to test an idea. Some of this exists in parts (like hubs in Toronto or Montreal), but extending it to more communities (leveraging colleges and regional development agencies) would broaden reach to SMEs in smaller cities and rural areas.
Monitoring and Adjusting: Finally, policy measures should be accompanied by ongoing measurement of adoption rates and barriers (much like the surveys and studies we’ve cited). The government, perhaps through Statistics Canada or the Business Development Bank, should regularly survey SMEs about their use of AI and what’s preventing it. This data-driven approach ensures that initiatives can be tweaked for effectiveness. For example, if in two years the data shows awareness has improved but financing is still a big issue, then more financial incentive programs can be rolled out or adjusted.
Collectively, these ecosystem and policy actions create a supportive environment for SMEs. Canada’s approach of combining investment, talent development, and responsible AI leadership (through organizations like GPAI, the global AI partnership, which Canada co-chairs) sets a positive tone. The key is to translate high-level strategy into on-the-ground impact for the average small business. By aligning public policy with the needs of SMEs – making it easier for them to try and adopt AI – Canada can close the adoption gap while upholding the values of trust and responsibility in AI.
6. Embracing Incremental Adoption and Innovation Mindset
A final practical suggestion for SMEs themselves is to adopt an incremental, experimentative mindset towards AI. This isn’t so much a policy as a recommendation based on observed success patterns. Small and medium businesses should be encouraged to take small steps rather than seeing AI as a giant leap. This could mean starting with AI features already present in software they use (for example, fully utilizing the AI-driven analytics in their accounting or CRM system), then gradually moving to more custom applications as comfort grows. SMBs could also start with low-risk areas: e.g., using AI for internal process automation or decision support, where mistakes are tolerable, before deploying AI on customer-facing or mission-critical tasks.
Adopting an “agile” approach – trying something, learning, and iterating – tends to work well. Many experts suggest the idea of “pilot, then scale” for AI adoption. Concretely, an SME might pilot a chatbot on their website to answer FAQs (a relatively contained use case). If it works, great – they free up some customer service time. If it fails, the damage is limited and they’ve learned something for the next attempt. Over time these small wins can add up. Businesses should also document and measure outcomes of these experiments so they can build their own case studies. This creates internal buy-in (“we tried X and it saved Y hours or dollars”).
Another important aspect is to leverage off-the-shelf solutions as much as possible initially. There is an ever-growing range of AI tools that do not require deep technical integration – from AI-enhanced marketing tools to inventory management plugins. SMEs should be somewhat conservative about embarking on completely custom AI development (which can be costly and complex) unless they have a very clear use case and support. Instead, using ready-made solutions or light-weight AI APIs can deliver value quickly. Many cloud service providers offer AI services on a pay-per-use basis, which aligns well with the limited budgets of SMEs – they can pay a few cents per prediction or per document processed, scaling up usage as needed rather than investing in full infrastructure upfront.
Lastly, celebrating and learning from progress is vital. When an SME successfully adopts an AI solution, it should share that story (with employees, in its industry network, maybe even with media if notable). This not only reinforces the positive culture internally but contributes to the broader ecosystem of shared learning. As Canada builds a tapestry of such stories from various sectors – a farm cooperative using AI to forecast yields, a local logistics firm using AI for route optimization, a chain of bakeries using AI to predict demand – the concept of AI in small business will become normalized.
In conclusion, the challenges to AI adoption by Canadian SMBs are real but not insurmountable. With the right mix of education, incentives, skilled people, cultural openness, and supportive policy, these barriers can be significantly lowered. Canada is already moving in this direction: investments are being made and awareness is rising. The payoff for overcoming these obstacles will be substantial – AI-adopting SMEs can become more efficient, competitive, and innovative, which in aggregate boosts Canada’s productivity and prosperity. As one recent report noted, widespread AI adoption could add between 1% and 6% to Canada’s productivity growth over the next decade, a much-needed boost at a time of sluggish performance. Moreover, by ensuring SMEs across all sectors and regions are included in the AI wave, Canada can avoid a digital divide and harness diverse talent and ideas in the AI era. The next few years (2024–2025 and beyond) will be critical in translating strategies into action. The momentum from generative AI’s popularity can be channeled into lasting digital transformation for SMEs. By tackling the primary barriers – from knowledge and cost to talent and trust – Canada’s small and medium businesses can confidently step into the AI age, unlocking new opportunities for themselves and the economy at large.
Sources
BDC, “The AI Imperative for Canada’s Entrepreneurs” (2024) – Survey of 1,247 business owners on AI adoption (New BDC study reveals 27% of Canadian entrepreneurs don’t know they’re using artificial intelligence (AI) | BDC.ca) (New BDC study reveals 27% of Canadian entrepreneurs don’t know they’re using artificial intelligence (AI) | BDC.ca)
Statistics Canada / The Dais, “Automation Nation? AI Adoption in Canadian Businesses” (2023) – Analysis of AI adoption rates and barriers using StatsCan data (Automation Nation? AI Adoption in Canadian Businesses) (Automation Nation? AI Adoption in Canadian Businesses)
Canadian Chamber of Commerce, “Prompting Productivity: Generative AI Adoption by Canadian Businesses” (2024) – Findings on generative AI uptake, trust, and challenges (Research Money Inc.) (Research Money Inc.)
Innovating Canada (W. Cukier), “AI Adoption Strategy for Canadian Business is Essential” (2024) – Commentary on Canada’s AI paradox and SME challenges (New Report Shows AI Adoption Strategy for Canadian Business is Essential - Innovating Canada) (New Report Shows AI Adoption Strategy for Canadian Business is Essential - Innovating Canada)
Forbes (H. Jones), “Inside Canada’s $2.4B Bet to Close the AI Adoption Gap” (Nov 2024) – Insights into Canada’s AI adoption gap and government initiatives (Inside Canada’s $2.4 Billion Bet To Close The AI Adoption Gap | Hessie Jones) (Inside Canada’s $2.4 Billion Bet To Close The AI Adoption Gap | Hessie Jones)
Government of Canada (ISED) News Release, “Federal government launches programs to help SMEs adopt AI solutions” (Oct 22, 2024) – Details on RAII and AI Assist funding for SME adoption (Federal government launches programs to help small and medium-sized enterprises adopt and adapt artificial intelligence solutions - Canada.ca)
Deloitte Canada, “Impact and Opportunities: Canada’s AI Ecosystem 2023” – Survey of organizations on AI adoption, with focus on SMEs and barriers (Impact and opportunities: Canada's AI ecosystem - 2023) (Impact and opportunities: Canada's AI ecosystem - 2023)
CDW Canada, “2024 Canadian AI and Data Analytics Trends Report” – Survey of IT decision-makers highlighting confidence and ethical concerns (2024 Canadian AI and Data Analytics Report | CDW Canada) (2024 Canadian AI and Data Analytics Report | CDW Canada)
Research Money Inc., “Canadian businesses lagging in adopting generative AI” (May 2024) – Cites Statistics Canada data on adoption rates and global comparison (Research Money Inc.) (Research Money Inc.).